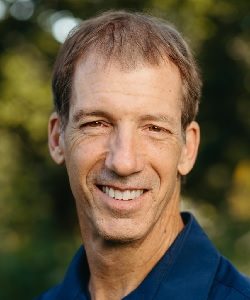
Professor of Psychology John Curtin has received a $3.2 million grant from the National Institutes of Health to develop a mobile app-based engagement system, guided by machine learning, to help individuals with alcohol use disorder stay engaged and improve their recovery outcomes.
For several years, Curtin’s Addiction Research Center has been developing systems to monitor and predict recovery patterns in participants with alcohol or opioid use disorders over extended periods. By tracking a range of daily activities, including self-reported experiences, GPS data, and voice/text communications, researchers can predict with remarkable accuracy when a participant is likely to lapse back into substance use.
“We can predict if someone is at risk of relapsing in the next week, day, or even hour,” says Curtin. “Not only can we predict when a lapse may happen, but we can also use these same models to figure out why they’re likely to lapse.”
This new grant will advance that work by exploring how these predictions can actively benefit participants. The study will involve 416 individuals recovering from alcohol use disorder, all of whom are one to eight weeks into abstinence. Their progress will be monitored over a six-month period, with data collection extending over three years.
Each night, researchers will use the machine learning model to predict the next day’s risk of lapse for each participant, identifying key factors driving that risk. The model will determine whether a participant is at high or low risk for using the following day, whether their risk has shifted in the past two weeks, and what is contributing to the risk (e.g., reported cravings, self-efficacy for maintaining abstinence, stress). Using the Multiphase Optimization Strategy (MOST), researchers will send messages providing personalized and relevant information about their risk, including recommendations for intervention or support, based on predictions from the machine learning model.
“We’re also focused on ensuring participants trust the system,” Curtin notes. “Without trust, the system can’t help them. We’ll be evaluating what types of information increase their trust and what they find most helpful, ensuring it delivers real clinical benefits.”